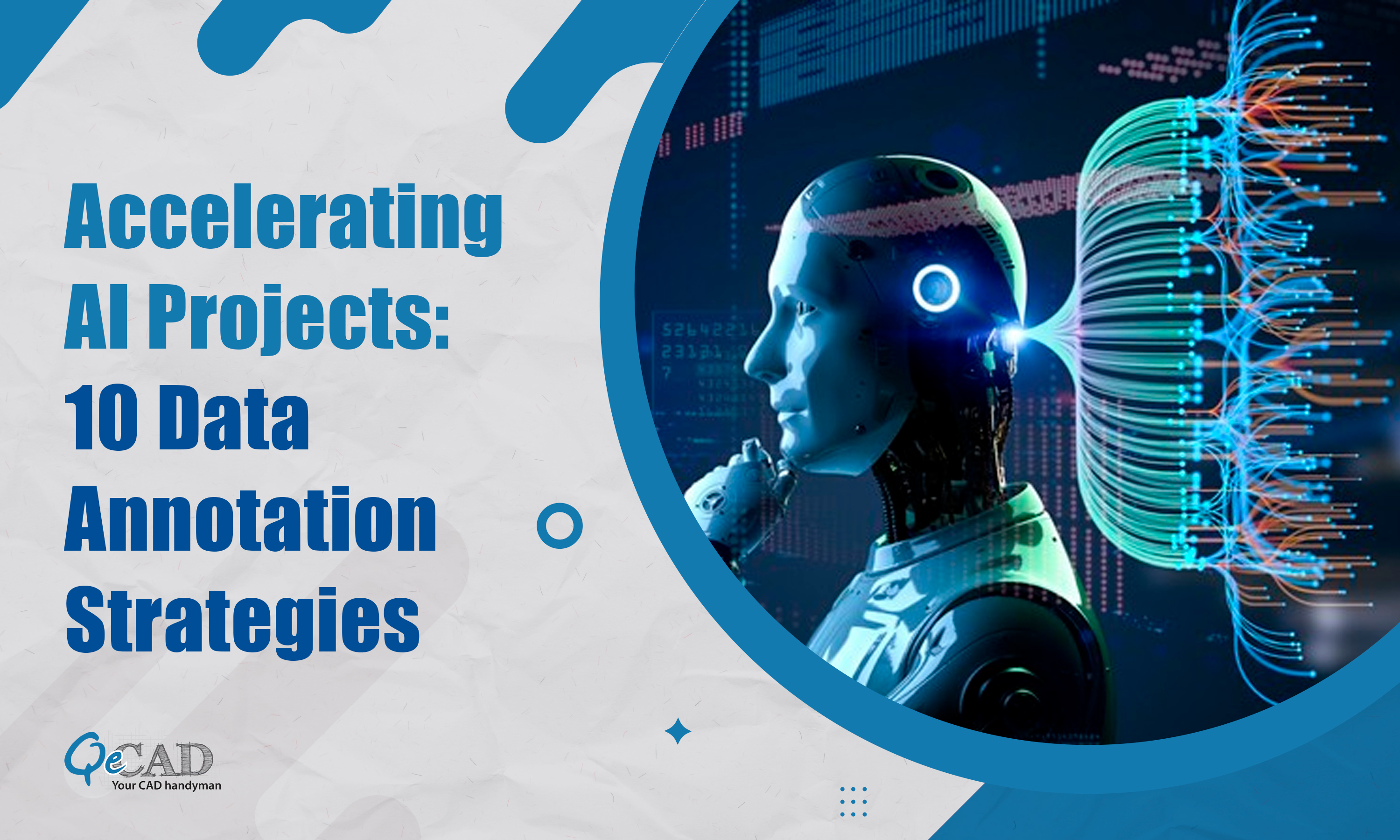
Introduction
In the rapidly evolving landscape of artificial intelligence (AI), the importance of high-quality annotated data cannot be overstated. Data annotation is a crucial step in the success of AI projects, involving the labelling of data to train machine learning models. The accurate labelling of data, facilitated through data annotation, contributes to the development of intelligent systems that can enhance and complement CAD Drafting Services in various industries. It forms the basis on which algorithms acquire the ability to identify patterns and make informed decisions. In this blog post, we will indulge in various data annotation strategies that can significantly accelerate your AI projects.
Top 10 Data Annotation Strategies Boost Your AI Projects
1) Understanding the Importance of Data Annotation
Before diving into strategies, it’s crucial to recognize why data annotation is a cornerstone of AI development. Annotated data serves as a guide for machine learning models, helping them learn and generalize patterns. The performance of the model is directly influenced by the quality of annotated data, whether it’s in the domains of image recognition, natural language processing, or object detection. Data annotation becomes essential to train machine learning models that can enhance the capabilities of Construction Documentation Services. This might include annotating data related to architectural styles, structural elements, or design specifications.
2) Choose the Right Annotation Types
Different AI projects require different types of annotations. For image-based projects, bounding boxes, polygons, and key points might be essential, while text-based projects may require entity recognition or sentiment analysis. Understanding the specific needs of your project is crucial for selecting the right annotation types.
3) Leverage Automated Annotation Tools
To accelerate the data annotation process, consider leveraging automated annotation tools. These tools use pre-trained models to suggest annotations, significantly reducing the manual workload. While they may not be flawless, combining automated tools with human annotators can enhance efficiency without compromising accuracy.
4) Crowdsourcing for Scale
Crowdsourcing platforms provide a cost-effective and scalable solution for data annotation. Platforms like Amazon Mechanical Turk or specialized annotation services allow you to tap into a large pool of annotators to label your data swiftly. Ensure clear instructions and quality checks to maintain annotation accuracy.
5) Active Learning for Iterative Improvement
Implementing an active learning strategy allows your model to iteratively improve its performance. Train your model on a subset of annotated data, identify areas of uncertainty, and prioritize annotating those instances. This iterative process refines the model’s understanding, resulting in enhanced overall performance.
6) Consistency is Key
Ensuring consistency in data annotation is essential for effective model training. Develop clear annotation guidelines and ensure that all annotators follow them meticulously. Frequent quality assessments and feedback loops can effectively address inconsistencies, contributing to an improved overall quality of annotated data.
7) Transfer Learning for Small Datasets
In scenarios where acquiring a large labelled dataset is challenging, transfer learning can be a game-changer. Pre-trained models on massive datasets can be fine-tuned with smaller, domain-specific datasets. This strategy proves advantageous, especially in situations where resources are constrained.
8) Implementing Active Feedback Loops
Establishing active feedback loops between annotators and model developers is essential for continuous improvement. Annotators can provide insights into challenging cases, helping developers refine models and update annotation guidelines. This iterative process ensures that the model evolves with the complexity of the data.
9) Quality Assurance Measures
Quality assurance is paramount in data annotation. Implementing a robust quality assurance process involves regular audits, double-checking annotations, and providing constructive feedback to annotators. This ensures the reliability of annotated data and contributes to the overall success of the AI project.
10) Security and Privacy Considerations
When outsourcing data annotation, prioritize security and privacy. Annotators often deal with sensitive information, and it’s crucial to establish protocols that safeguard data integrity. Non-disclosure agreements and secure communication channels should be in place to protect both the annotated data and the client’s information.
Conclusion
Accelerating AI projects through effective data annotation strategies requires a combination of careful planning, leveraging technology, and maintaining a focus on quality. Whether you opt for automated tools, crowdsourcing, or a hybrid approach, the key lies in understanding the unique requirements of your AI project. By implementing these strategies, you can streamline the data annotation process, leading to faster model development, improved accuracy, and ultimately, the success of your AI initiatives.